The major driver behind Machine Learning going mainstream is the exponential growth of chip performance through Moore’s law over the last four decades. However, when it comes to ML for the future, Moore’s law is not enough. Mere scaling through going to a lower node is not sufficient to keep pace with the ever-increasing complexity of ML workloads for both training and inference. Add energy efficiency to the mix and we need a dramatically new class of hardware – silicon optimized for specific domains. And this new class of hardware requires a new paradigm of system and silicon design.
Two technologies that sit at the heart of this new paradigm are High Level Synthesis (HLS) and Embedded Analytics. By raising the abstraction level of hardware design, HLS enables HW/SW co-design for the ML accelerator. Chip architects can quickly converge on partitioning the functionality between software running on the SoC’s embedded processor and optimal custom hardware. They can also optimize their SoC for the right balance of functionality, power, performance, and area. Embedded Analytics takes this principle even further. By adding visibility into the chip at any level of detail, it enables architects and designers to optimize the software load, and add even further degrees of differentiation to the SoC. By monitoring the chip’s behavior throughout its lifecycle, Embedded Analytics also provides valuable data that can be used for the next iteration of the hardware. By closing the loop and monitoring the performance of new Catapult HLS enabled architecture, Embedded Analytics gives users the unique ability to learn how the device performs during the prototype and validation phases and into field deployment.
In this webinar, we will share this new model of data driven hardware design which helps users produce dramatically better hardware and software – tightly optimized for specific applications.
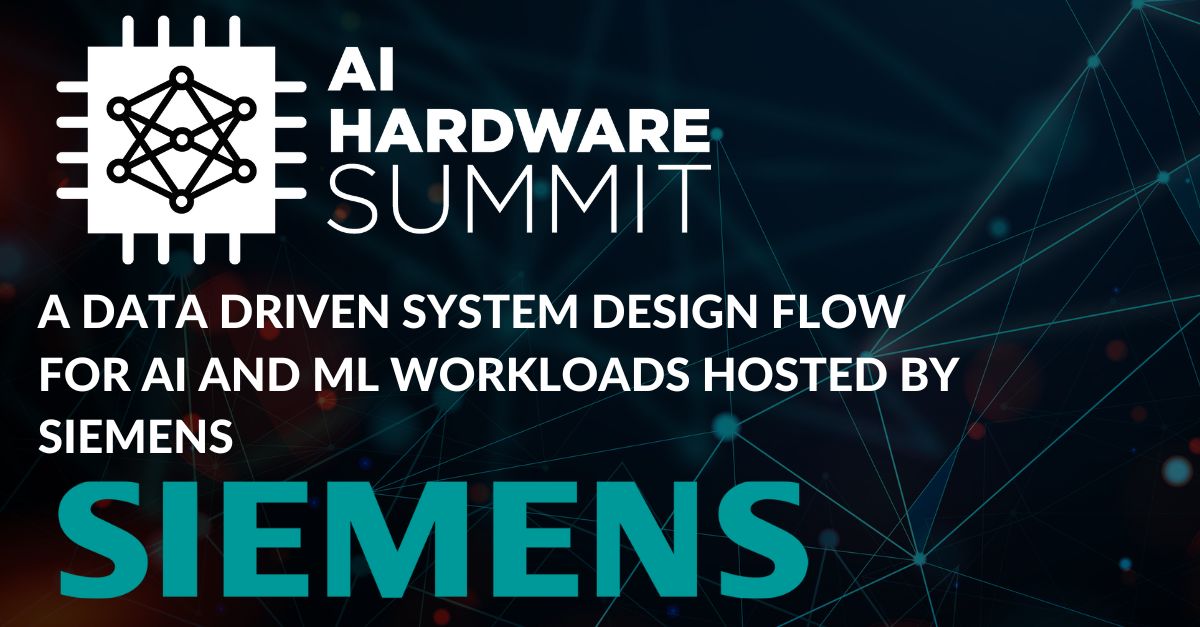